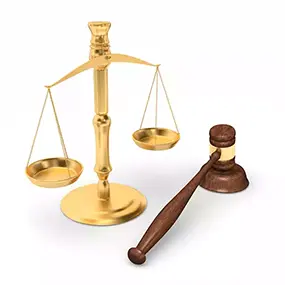
Fair Credit Reporting Act News
Automated credit reporting systems' algorithmic mistakes can compromise customer confidence in data-driven procedures
Sunday, December 8, 2024 - To handle and evaluate enormous volumes of consumer data, credit reporting has turned more and more to automated methods. Credit data is reported to the main bureaus--Equifax, Experian, and TransUnion--by algorithms that classify, update, and report. Automation brings new dangers, including algorithmic errors that could result in erroneous credit profiles and unjustifiable financial implications, even while it increases productivity and lowers hand-made errors. Pulling data from lenders, public records, and debt collectors among other sources, automated credit reporting systems operate. After that, this information is arranged and included in a credit report for a consumer. From data collecting to algorithmic classification, mistakes can happen at any level of this process. Typical errors include misclassifying accounts, neglecting to update payments, or mismatched data leading to the incorrect consumer profile. The Federal Trade Commission (FTC) estimates that one in five Americans have at least one credit report issue; automated systems are typically the cause of these mistakes. Concerns concerning automated credit reporting have also been raised by the Consumer Financial Protection Bureau (CFPB), which emphasizes that algorithmic vulnerabilities can allow systematic mistakes across millions of accounts to be sustained without timely discovery.
Algorithmic errors in credit reporting have potentially dire effects. For consumers, even little mistakes could cause significant financial losses. For example, a credit score may be much reduced if an algorithm misclassifies paid-off debt as delinquent. Higher interest rates refused loan applications, or lower credit limits might all follow from this. Worse still, many customers are not aware of these mistakes until they apply for credit, only to discover unwelcome terms or rejections. Additional systemic inequality are algorithmic mistakes. Making decisions in automated systems depends on past data, hence any errors or biases in the data could produce unjust results. For instance, if a customer from an underprivileged background has insufficient or outdated information in their financial profile, mistakes could disproportionately impact them. These errors can prolong already-existing differences in credit and financial resource availability. Dealing with these concerns calls for both consumer awareness and institutional changes. For individuals, routinely looking at credit records is crucial. Examining accounts, balances, and payment history carefully helps one find possible computational problems. Should an error be found, consumers should promptly open a dispute with the credit bureau. Credit bureaus under the Fair Credit Reporting Act (FCRA) have thirty days to look over conflicts and fix mistakes. Supporting records, such as letters to lenders or payment receipts, help a consumer's case be stronger. If there are ongoing mistakes, you might have to raise the matter to the CFPB or speak with a Fair Credit Reporting Act lawyer. Beyond personal action, structural adjustments are necessary to lower algorithmic errors. To increase the accuracy and openness of automated credit reporting systems, advocacy organizations such as the National Consumer Law Center (NCLC) have urged tougher rules. Among the suggested changes are mandated audits of credit reporting systems, more data source monitoring, and stronger consumer rights for contested mistakes.