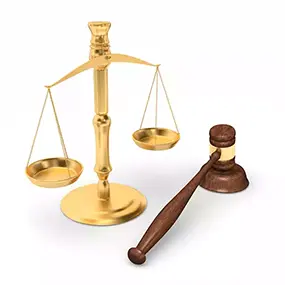
Fair Credit Reporting Act News
Exploring the advantages, difficulties, and consumer protection associated with computerized credit report-based lending choices
Wednesday, July 10, 2024 -
In the context of automated lending decisions in consumer credit, it is critical to comprehend credit report inaccuracies and file a Fair Credit Reporting Act complaint. The financial industry has undergone a transformation thanks to automated lending decision-making, which makes credit application processing faster and more effective. But there are also important ramifications for customers and lenders alike from this change, especially in terms of transparency, equity, and accuracy. Algorithms that evaluate credit report data to determine an applicant's creditworthiness are what power automated determinations. Due to these algorithms' ability to process enormous volumes of data quickly, lenders are able to provide loan judgments almost instantly. Lenders gain from this efficiency by cutting expenses associated with operations, while consumers gain from quicker credit availability. However, the quality of the information in credit reports is crucial to the accuracy of these judgments. Errors in credit reports may result in inaccurate evaluations, which may cause customers to face unfair lending terms or unfair denials. The elimination of human prejudice is one of the main advantages of automated lending decisions. When developed correctly, algorithms can evaluate creditworthiness using objective standards, reducing the possibility of discrimination on the basis of gender, color, or other non-financial characteristics. This advantage is dependent on the algorithms' own design as well as the accuracy and fairness of the data inputs. Automated systems have the potential to reinforce or even worsen preexisting disparities if they use biased data or incorrect algorithms.
In the context of automated decision-making, consumer protection under the FCRA has significant importance. When negative actions, such as credit denials, are made based on a customer's credit report, the FCRA mandates that the consumer be notified. The name of the CRA that supplied the report and the justifications for the adverse action must be mentioned in this notification. Because of this openness, customers can spot and challenge any errors in their credit reports, defending their rights and guaranteeing just treatment. Even with the benefits, computerized lending decisions come with a number of drawbacks. The decision-making process's opacity is one major cause for concern. Customers could become confused and frustrated if they can't figure out how certain things in their credit reports affect their choices. Sustaining customer confidence and regulatory compliance requires automated systems to give understandable, concise justifications for their actions. Data security is still another important concern. Large volumes of personal data are collected and processed by automated systems, which makes them appealing targets for hackers. To guard against security breaches and unwanted access to customer data, lenders need to put strong security measures in place. Furthermore, adhering to data privacy laws, such as the FCRA, is essential to avoiding legal troubles and preserving consumer trust.
Artificial intelligence (AI) and machine learning are becoming more and more important in automated lending choices. These tools can find trends and insights that traditional approaches would overlook, improving the efficiency and accuracy of credit assessments. However, the application of AI also brings up issues with transparency and accountability. Responsible implementation requires that decisions made by AI be comprehensible and that there be a well-defined process for resolving biases and mistakes. Lenders also need to think about how automated decision-making can affect financial inclusivity. Automation can expedite the loan process, but it can also bar people with non-traditional financial habits or insufficient credit history from applying. To reduce this risk and encourage greater inclusivity in lending, alternative credit assessment techniques that take a wider range of financial activities into consideration should be developed.